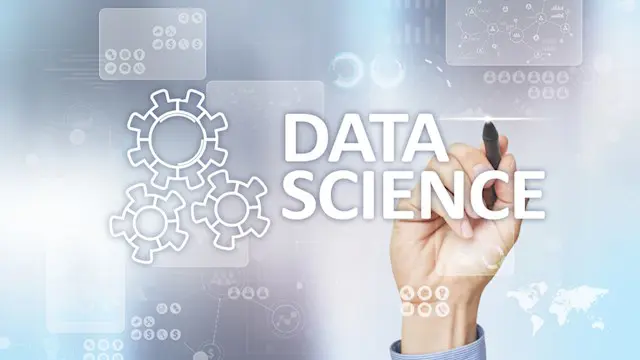
Data : Data Science online training
2 Courses Bundle | Free eCertificate |Data Science Masterclass | Tutor Support | Video Lessons
Frontier Education
Summary
- Reed courses certificate of completion - Free
- Tutor is available to students
Add to basket or enquire
Overview
This Data Science course includes a whole host of practical tips and advice, helping you to develop your data science skills to become the data scientist, data analyst or exiting careers in data science.
In this course you will learn Data Science and Machine Learning Models with R/ Python Programming Language. Machine learning techniques enable us to automatically extract features from data so as to solve predictive tasks, such as speech recognition, object recognition, machine translation, question-answering, anomaly detection, medical diagnosis and prognosis, automatic algorithm configuration, personalisation, robot control, time series forecasting, and much more.
This is bundle of two courses:
Course 1: Learn Data Science and Machine Learning with R from A-Z
Course 2: Learn Python for Data Science & Machine Learning from A-Z
Certificates
Reed courses certificate of completion
Digital certificate - Included
Will be downloadable when all lectures have been completed
Curriculum
-
DS & ML A-Z Course Intro 32:01
-
Getting started with R 44:52
-
Data Type and Structure in R 5:01:57
-
Intermediate R 4:14:11
-
Data Manipulation in R 5:39:59
-
Data Visualization in R 2:24:30
-
Creating-Reports-with-R-Markdown 28:54
-
Building Web Apps with R Shiny 1:31:28
-
Introduction to Machine Learning 1:08:35
-
Data Preprocessing 1:04:51
-
Linear Regression_A Simple Model 1:18:14
-
Exploratory Data Analysis 1:28:00
-
Linear Regression_A Real Model 1:24:52
-
Logistic Regression 1:17:26
-
Starting a career in data science 29:42
-
Python for Data Science 23:13:20
Course media
Description
With expert guidance and a combination of videos, PDFs, and worksheets, this course will enable you to develop your data cleaning, become a Data Analyst and unlock your full potential.
Implementation in R is done in such a way so that not only you learn how to implement a specific Model in Python but you learn how to build real times templates and find the accuracy rate of Models so that you can easily test different models on a specific problem, find the accuracy rates and then choose the one which give you the highest accuracy rate. This course is for you, if you are:
- Curious about learning Data Science and Machine Learning
- Curious to learn the Maths behind Machine Learning Models
- Interested in becoming a real time professional Data Scientist by knowing all the Maths and Intuition behind every Machine Learning Model
Curriculum of the courses:
Course 1: Learn Data Science and Machine Learning with R from A-Z
Section 1: Introduction to Data Science +ML with R from A-Z
Section 2: Getting Started with R
Section 3: Data Types and Structures in R
Section 4: Intermediate R
Section 5: Data Manipulation in R
Section 6: Data Visualisation in R
Section 7: Creating Reports with R Markdown
Section 8: Building Webapps with R Shiny
Section 9: Introduction to Machine Learning
Section 10: Data Preprocessing
Section 11: Linear Regression: A Simple Model
Section 12: Exploratory Data Analysis
Section 13: Linear Regression: A Real Model
Section 14: Logistic Regression
Section 15: Starting a Career in Data Science
Course 2: Learn Python for Data Science & Machine Learning from A-Z
Section 1: Introduction to Python for Data Science & Machine Learning from A-Z
Section 2: Data Science & Machine Learning Concepts
Section 3: Python For Data Science
Section 4: Statistics for Data Science
Section 5: Probability and Hypothesis Testing
Section 6: NumPy Data Analysis
Section 7: Pandas Data Analysis
Section 8: Python Data Visualisation
Section 9: Introduction to Machine Learning
Section 10: Data Loading & Exploration
Section 11: Data Cleaning
Section 12: Feature Selecting and Engineering
This Data Science course covers:
- Data Types and Structures in R : what you need to know
- Intermediate R: tailoring your approach to maximise impact
- Mastering data processing
- data manipulation
You’ll also be able to access a number of exclusive bonus resources to help you along your Data Science journey, including:
- Python For Data Science
- Statistics for Data Science
- NumPy Data Analysis
Career path
This Data Science is ideal for people looking to progress their career into a Data Scientist, for those who want to become a Data Analyst, as well as looking to further develop their skills and knowledge.
Questions and answers
Currently there are no Q&As for this course. Be the first to ask a question.
Reviews
Currently there are no reviews for this course. Be the first to leave a review.
Legal information
This course is advertised on Reed.co.uk by the Course Provider, whose terms and conditions apply. Purchases are made directly from the Course Provider, and as such, content and materials are supplied by the Course Provider directly. Reed is acting as agent and not reseller in relation to this course. Reed's only responsibility is to facilitate your payment for the course. It is your responsibility to review and agree to the Course Provider's terms and conditions and satisfy yourself as to the suitability of the course you intend to purchase. Reed will not have any responsibility for the content of the course and/or associated materials.